Контролируемый техпроцесс для прогнозирования литофаций в сложных неоднородных плотных песчаных коллекторах: Основанный на данных подход с использованием моделей кластеризации и классификации
Аннотация
Полный текст:
PDFЛитература
Afzal, J., Kuffner, T., Rahman, A., & Ibrahim, M. (2009). Seismic and Well-log Based Sequence Stratigraphy of The Early Cretaceous, Lower Goru “C” Sand of The Sawan Gas Field, Middle Indus Platform, Pakistan. Proceedings, Society of Petroleum Engineers (SPE). Pakistan Association of Petroleum Geoscientists (PAPG) Annual Technical Conference, Islamabad, Pakistan.
Akkurt, R., Conroy, T. T., Psaila, D., Paxton, A., Low, J., & Spaans, P. (2018). Accelerating and Enhancing Petrophysical Analysis With Machine Learning: A Case Study of an Automated System for Well Log Outlier Detection and Reconstruction. SPWLA 59th Annual Logging Symposium, 2-6 June, London, UK.
Alghazal, M., & Krinis, D. (2021). A novel approach of using feature-based machine learning models to expand coverage of oil saturation from dielectric logs. Society of Petroleum Engineers -SPE Europec Featured at 82nd EAGE Conference and Exhibition, EURO 2021, 2, 10. https://doi.org/10.2118/205162-ms
Ali, M., Jiang, R., Ma, H., Pan, H., Abbas, K., Ashraf, U., & Ullah, J. (2021). Machine learning - A novel approach of well logs similarity based on synchronization measures to predict shear sonic logs. Journal of Petroleum Science and Engineering, 203, 108602. https://doi.org/10.1016/ j.petrol.2021.108602
Ali, M., Khan, M. J., Ali, M., & Iftikhar, S. (2019). Petrophysical analysis of well logs for reservoir evaluation: a case study of “Kadanwari” gas field, middle Indus basin, Pakistan. Arabian Journal of Geosciences, 12(6). https://doi.org/10.1007/s12517-019-4389-x
Ali, M., Ma, H., Pan, H., Ashraf, U., & Jiang, R. (2020). Building a rock physics model for the formation evaluation of the Lower Goru sand reservoir of the Southern Indus Basin in Pakistan. Journal of Petroleum Science and Engineering, 194,107461. https://doi.org/10.1016/j.petrol.2020.107461
Antariksa, G., Muammar, R., & Lee, J. (2022). Performance evaluation of machine learning-based classification with rock-physics analysis of geological lithofacies in Tarakan Basin, Indonesia. Journal of Petroleum Science and Engineering, 208, 109250. https://doi.org/https://doi.org/10.1016/j.petrol.2021.109250
Ashraf, U., Zhang, H., Anees, A., Ali, M., Zhang, X., Shakeel Abbasi, S., & Nasir Mangi, H. (2020). Controls on Reservoir Heterogeneity of a Shallow Marine Reservoir in Sawan Gas Field, SE Pakistan: Implications for Reservoir Quality Prediction Using Acoustic Impedance Inversion. Water, 12(11). https://doi.org/10.3390/w12112972
Berger, A., Gier, S., & Krois, P. (2009). Porosity-preserving chlorite cements in shallow marine volcaniclastic sandstones: Evidence from cretaceous sandstones of the sawan gas field, Pakistan. AAPG Bulletin. https://doi.org/10.1306/01300908096
Chawshin, K., Gonzalez, A., Berg, C. F., Varagnolo, D., Heidari, Z., & Lopez, O. (2021). Classifying Lithofacies from Textural Features in Whole Core CT-Scan Images. SPE Reservoir Evaluation & Engineering, 24(02), 341–357. https://doi.org/10.2118/205354-PA
Guresen, E., & Kayakutlu, G. (2011). Definition of Artificial Neural Networks with comparison to other networks. Procedia Computer Science, 3, 426–433. https://doi.org/10.1016/j.procs.2010.12.071
Guyon, I., & Elisseeff, A. (2003). An Introduction to Variable and Feature Selection. J. Mach. Learn. Res., 3(null), 1157–1182.
Haykin, S. O. (2011). Neural Networks and Learning Machines. Pearson Education. https://books.google.com/books?id=faouAAAAQBAJ
Kazmi, A. H., & Jan, M. Q. (1997). Geology and tectonics of Pakistan. Graphic publishers.
Li, Y., Li, T., & Liu, H. (2017). Recent advances in feature selection and its applications. Knowledge and Information Systems, 53(3), 551–577. https://doi.org/10.1007/s10115-017-1059-8
Song, L., Liu, Z., Li, C., Ning, C., Hu, Y., Wang, Y., Hong, F., Tang, W., Zhuang, Y., Zhang, R., & others. (2021). Prediction and Analysis of Geomechanical Properties of Jimusaer Shale Using a Machine Learning Approach. SPWLA 62nd Annual Logging Symposium.
Valentín, M. B., Bom, C. R., Coelho, J. M., Correia, M. D., de Albuquerque, M. P., de Albuquerque, M. P., & Faria, E. L. (2019). A deep residual convolutional neural network for automatic lithological facies identification in Brazilian pre-salt oilfield wellbore image logs. Journal of Petroleum Science and Engineering, 179, 474–503. https://doi.org/https://doi.org/10.1016/j.petrol.2019. 04.030
Valzania, S., Kfoury, M., Grandis, M., Valdisturlo, A., Fanello, G., Guerra, L., Heikal, S., Kashif, A., & Sultan, A. (2011). Kadanwari field: A tight gas reservoir study and a successful pilot well give new life to an exploited field. 73rd European Association of Geoscientists and Engineers Conference and Exhibition 2011: Unconventional Resources and the Role of Technology. Incorporating SPE EUROPEC 2011, 4, 2715–2744. https://doi.org/10.2118/143001-ms
Vapnik, V. N. (1995). The Nature of Statistical Learning Theory. Springer-Verlag. https://doi.org/https://doi.org/10.1007/978-1-4757-3264-1
Zhang, J., Ambrose, W., & Xie, W. (2021). Applying convolutional neural networks to identify lithofacies of large-n cores from the Permian Basin and Gulf of Mexico: The importance of the quantity and quality of training data. Marine and Petroleum Geology, 133, 105307. https: //doi.org/10. 1016/ j.marpetgeo.2021.10530
DOI: http://dx.doi.org/10.17072/psu.geol.22.4.342
Ссылки
- На текущий момент ссылки отсутствуют.

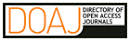

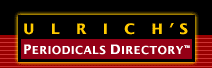
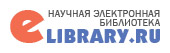

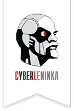
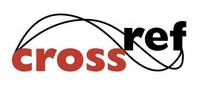
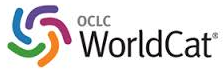
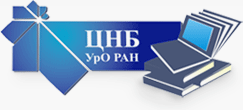
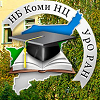
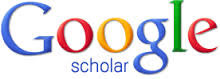
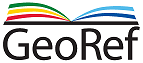